Published on: 2023-08-23
Written by the Callan Abrahams
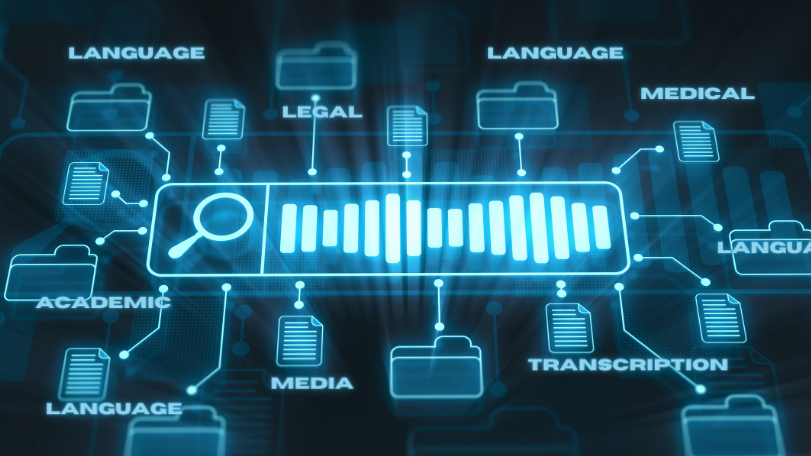
Enlabeler will be embarking on an enlightening journey into the world of Automatic Speech Recognition (ASR) model refinement. ASR, the transformative technology that converts spoken language into text, has revolutionized industries ranging from transcription services to voice assistants. However, the path to achieving accurate and unbiased transcriptions is not without its challenges.
ASR models, as remarkable as they are, require meticulous tuning to ensure they accurately capture the nuances of human speech across diverse contexts, accents, and languages. This series is designed to be your guide through the intricate process of ASR model fine-tuning.
We start by discussing a critical challenge that takes centre stage: BIAS. This article discusses advanced bias mitigation techniques, equipping you to ensure your ASR models transcribe speech with impartiality, fostering diversity and respect for all voices.
ASR, while revolutionary, faces a crucial hurdle – unintentional bias. ASR models might favour certain demographics, misrepresent speech, or even amplify societal prejudices. This bias challenge is more than just a technical issue; it’s an ethical imperative that calls for advanced solutions. Our mission? To provide you with the tools and insights to confront and conquer bias head-on. Let’s explore strategies that empower your ASR models to transcribe speech while adhering to principles of fairness and diversity.
‘I think unconscious bias is one of the hardest things to get at.’ – Ruth Bader Ginsburg
Before addressing bias, identification is key. Advanced techniques like disparity analysis and fairness metrics serve as your guiding light. These methods shine a spotlight on discrepancies in the treatment of different groups within your training data, empowering you to address the problem head-on. Imagine a dinner party with diverse voices; each deserves an equal seat at the table. Rebalancing techniques ensure that underrepresented groups receive the attention they deserve. By augmenting or oversampling these groups, you level the playing field, ensuring your model’s transcriptions respect all voices equally.
Oversampling involves intentionally increasing instances of the minority class, promoting balanced representation and reducing bias towards the more common class.
Rebalancing techniques in ASR involve giving extra attention to underrepresented groups or words in your training data. This ensures fair and accurate transcriptions by exposing the model to diverse voices and words.
In the quest for fairness, adversarial debiasing emerges as a formidable strategy. This is a challenge that many AI/ML-driven systems struggle to address. Here, your model is trained not only to recognize speech but also to differentiate between genuine content and biassed content. This dual-purpose training encourages the model to adopt a neutral stance, eradicating unintentional biases. By explicitly identifying and mitigating biases during training, adversarial debiasing enhances the model’s ability to provide impartial transcriptions. After the initial ASR transcriptions, the journey isn’t over. Post-processing is your opportunity to refine and rectify. Leveraging techniques like re-ranking, you can modify the transcriptions to ensure a fair representation of all speech patterns, removing any unintentional distortions.
Human-AI Collaboration: The Final Verdict
In the battle against bias, humans and AI stand united. Human reviewers play a vital role in detecting nuanced biases that automated processes might miss. By incorporating their insights into the fine-tuning loop, you create a dynamic, ongoing process that continuously improves model fairness. Ultimately, bias mitigation is not just a strategy—it’s a pledge to ethical AI development. Armed with these advanced techniques, you’re poised to create ASR models that transcend biases, offering accurate transcriptions that reflect the richness and diversity of human speech.
So, fellow champions of fairness, let’s embrace these techniques and ensure our ASR models illuminate the world with transcriptions that are free from prejudice. Remember, every word matters and every voice deserves to be heard.
Contact us at [email protected] to discuss how we can assist you with your ASR fine-tuning model across different minority languages and domains.